
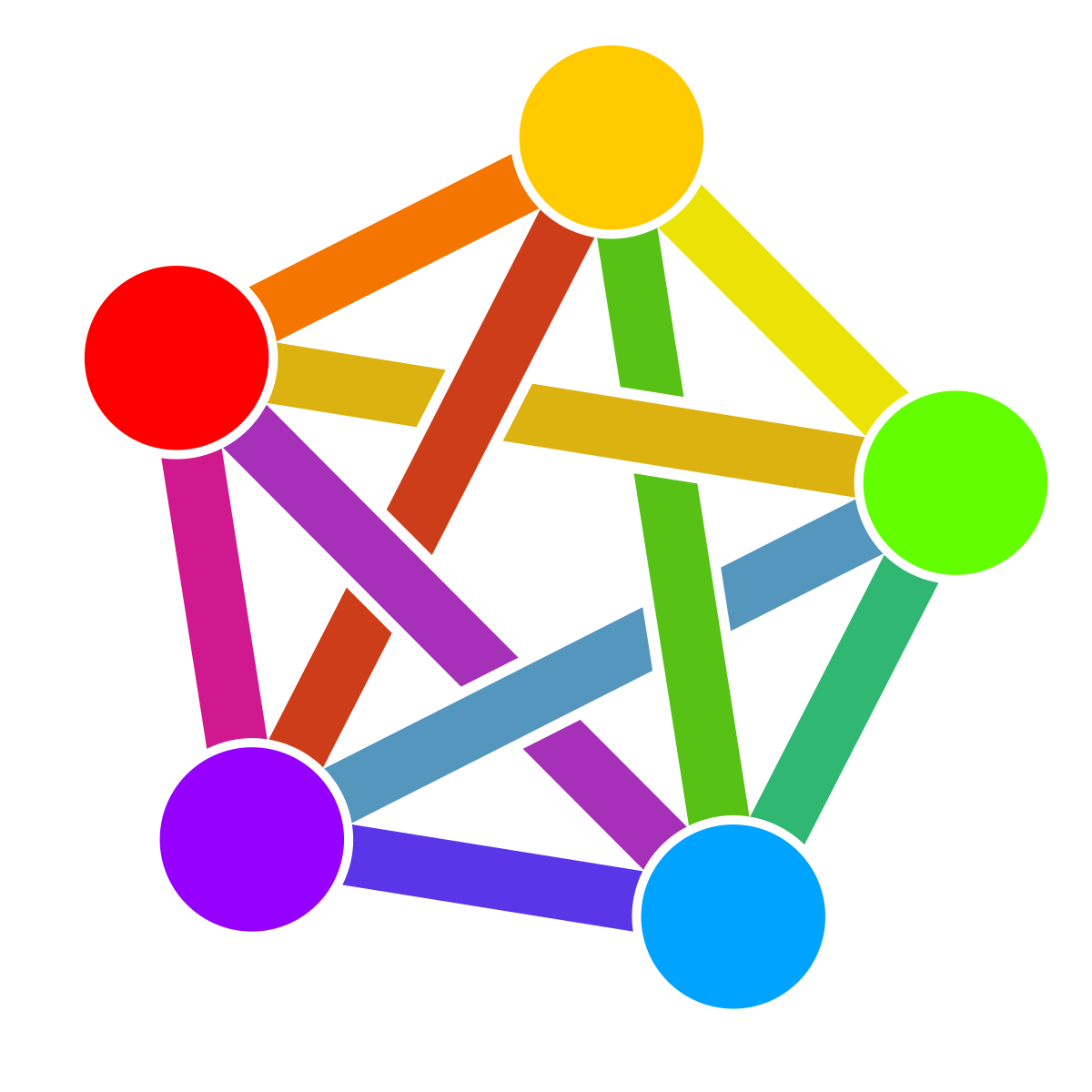
Thing is it’s kinda too late, and the, uh, “commercial net” has all but taken over society.
Whatever happens, it would be nice if that part burns down. And I think yanking the techies from the space with the Fediverse will help.
Thing is it’s kinda too late, and the, uh, “commercial net” has all but taken over society.
Whatever happens, it would be nice if that part burns down. And I think yanking the techies from the space with the Fediverse will help.
At risk of rambling… It feels like attention spans have shortened, too.
https://www.axios.com/2024/11/29/gen-z-kids-reading-tv-songs
People don’t want to dig through long discussions and documentation, they want a quick fix in a YouTube Short, or for it to be fed to them shooting the breeze in Discord.
And this sorta works short term, until the “old” information well those shorter systems rely on dries up.
It’s already a serious problem in newer topics. I’m part of the “localllama” community, for instance, and it feels like any central organization of knowledge has completely collapsed, and there is no old info to fall back on because everything is so new.
Anything anti billionaire, to sum it up.
Yeah, well, Facebook, Twitter, YouTube, TikTok are not the net, they are siloes. Discord too. Even Reddit is trying as hard as it can to be insular.
Much of my family doesn’t even know how to use a browser, at least not beyond the bare minimum for work. They probably never will.
I think old school internet folks are underestimating just how much of a grip Big Tech has on users’ attention.l, and their devices.
Discord is scary popular though, like Facebook popular. I am really scared the enshittification will stick hard, like it has for Facebook.
let the world burn
That’s what’s gonna happen.
Maybe Europe and China will “isolate” themselves from much of the burning. I hope they do. But the rest of the world seems quite entrenched in Big Tech.
Maybe burning quickly is better, since more people will notice.
You mean drag them from platforms that have a vested interest in keeping them locked in and squashing competitors like the Fediverse?
In platforms that spend billions on engagement optimization algorithms, with the sole purpose of keeping users addicted, basically with government and business landscape backing?
Look, I’m optimistic about the Fediverse, this is a great refuge in the hellscape that is the internet. But you can’t make people want to change. I’ve learned this IRL, but see it with (for example) persecuted people continuing to use Twitter even though its owner basically has a gun to their heads. There’s a big gulf between being a fantastic refuge and taking the internet from Facebook and Google. Even if every phone on the planet had an easy button to switch to Fediverse alternatives in one click… many would not take it, and that’s an utter fantasy.
Or Matrix?
According to history:
Wait till it’s so enshittified it’s unusable, or…
If it reaches a critical mass… You can’t. See: Facebook.
The Fediverse can adopt a few nice communities, but honestly bringing the larger population seems hopeless.
Today. Among thousands of times.
I’m with OP. People have been screaming this for ages, and the collective societal reaction hasn’t even been apathy, but “We vote for Big Tech CEOs, full steam ahead.”
So… Yeah, I’m tired, too. Screw it all. Let the internet burn in Reddit/Discord/SEO hell. Maybe we can build something from the ashes.
Ugh, Discord is an information black hole. I despise how so many of my niches have fled there.
Reddit seems to be trying to destroy that “role” of theirs as hard as they can though. A few very niche subs I follow are drying from some kind of “bug” that deprioritizes their discoverability.
It’s not a bug. It’s absolutely a feature for making Reddit more generic, farmable garbage and noise.
A problem is volunteers and critical mass.
Open source “hacks” need a big pool of people who want something to seed a few brilliant souls to develop it in their free time. It has to be at least proportional to the problem.
This kinda makes sense for robot vacuums: a lot of people have them, and the cloud service is annoying, simpler, and not life critical.
Teslas are a whole different deal. They are very expensive, and fewer people own them. Replicating even part of the cloud API calls is a completely different scope. The pool of Tesla owners willing to dedicate their time to that is just… smaller.
Also, I think buying a Tesla, for many, was a vote of implicit trust in the company and its software. It’s harder for someone cynical of its cloud dependence to end up with an entire luxury automobile.
Open source serving algorithms exist. They have the benefit of being customizable, not purely engagement driven.
As for tagging, I think Ao3’s system is exemplary: https://fanlore.org/wiki/AO3_Tagging_System
If it integrates with the fediverse, it can be promoted on other platforms and doesn’t need critical mass.
That’s the advantage. All the platforms are trying to synergize, not steal from each other like the corporate apps.
Yep.
I feel the fediverse should lean towards “overly aggressive” when combatting spam, before it takes root, even with all the negatives that brings.
To go into more detail:
Exllama is faster than llama.cpp with all other things being equal.
exllama’s quantized KV cache implementation is also far superior, and nearly lossless at Q4 while llama.cpp is nearly unusable at Q4 (and needs to be turned up to Q5_1/Q4_0 or Q8_0/Q4_1 for good quality)
With ollama specifically, you get locked out of a lot of knobs like this enhanced llama.cpp KV cache quantization, more advanced quantization (like iMatrix IQ quantizations or the ARM/AVX optimized Q4_0_4_4/Q4_0_8_8 quantizations), advanced sampling like DRY, batched inference and such.
It’s not evidence or options… it’s missing features, thats my big issue with ollama. I simply get far worse, and far slower, LLM responses out of ollama than tabbyAPI/EXUI on the same hardware, and there’s no way around it.
Also, I’ve been frustrated with implementation bugs in llama.cpp specifically, like how llama 3.1 (for instance) was bugged past 8K at launch because it doesn’t properly support its rope scaling. Ollama inherits all these quirks.
I don’t want to go into the issues I have with the ollama devs behavior though, as that’s way more subjective.
It’s less optimal.
On a 3090, I simply can’t run Command-R or Qwen 2.5 34B well at 64K-80K context with ollama. Its slow even at lower context, the lack of DRY sampling and some other things majorly hit quality.
Ollama is meant to be turnkey, and thats fine, but LLMs are extremely resource intense. Sometimes the manual setup/configuration is worth it to squeeze out every ounce of extra performance and quantization quality.
Even on CPU-only setups, you are missing out on (for instance) the CPU-optimized quantizations llama.cpp offers now, or the more advanced sampling kobold.cpp offers, or more fine grained tuning of flash attention configs, or batched inference, just to start.
And as I hinted at, I don’t like some other aspects of ollama, like how they “leech” off llama.cpp and kinda hide the association without contributing upstream, some hype and controversies in the past, and hints that they may be cooking up something commercial.
Yeah, so many projects and companies using Discord for support seemed like such a bad idea.